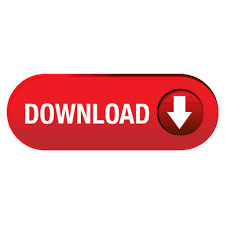

Stimulated by the Duan-Lukin-Cirac-Zoller protocol, many improved quantum repeater protocols based on quantum memories have been proposed, which commonly focus on the entanglement-distribution rate. Quantum repeaters are critical components for distributing entanglement over long distances in presence of unavoidable optical losses during transmission. Here we survey the cutting edge of this merger and list several open problems. Further, we are witnessing the emergence of a physical theory pinpointing the fundamental and natural limitations of learning. Progress has been rapid, fostered by demonstrations of midsized quantum optimizers which are predicted to soon outperform their classical counterparts. The most notable examples include quantum enhanced algorithms for principal component analysis, quantum support vector machines, and quantum Boltzmann machines.

On the other hand, quantum mechanics offers tantalizing prospects to enhance machine learning, ranging from reduced computational complexity to improved generalization performance. Traditional machine learning has dramatically improved the benchmarking and control of experimental quantum computing systems, including adaptive quantum phase estimation and designing quantum computing gates. Recent progress implies that a crossover between machine learning and quantum information processing benefits both fields. We provide the strengths and limitations of these algorithms and a novel literature survey that includes their use besides face detection. We present detailed comparisons among the algorithms in all-inclusive and under sub-branches. In our study, however, we cover detailed technical explanations of face detection algorithms and various recent sub-branches of the neural network. Earlier survey papers on face detection algorithms are limited to just technical details and popularly used algorithms.
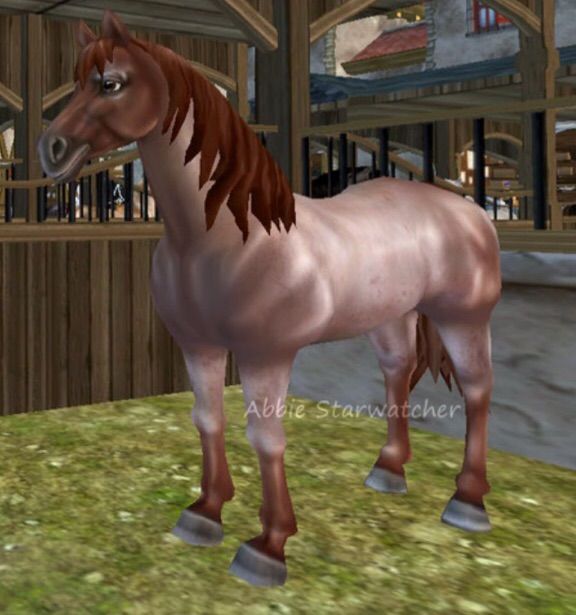
Lastly, we conclude this study with several promising research directions to pursue. Thirdly, we provide detailed comparisons among the algorithms epitomized to have an all-inclusive outlook. Secondly, we include a comparative evaluation among different algorithms in each single method. First, we explore a wide variety of the available face detection algorithms in five steps, including history, working procedure, advantages, limitations, and use in other fields alongside face detection. This paper aims at providing fourfold discussions on face detection algorithms. However, there is little attention paid in making a comprehensive survey of the available algorithms. Many astutely developed algorithms have been proposed to detect faces. The recent veer proliferation of computational resources is paving the way for frantic advancement of face detection technology. Our fully quantum pattern recognition system with quantum algorithm and quantum inputs promises a much-improved image acquisition and identification system with potential applications extending beyond face recognition, e.g., in medical imaging for diagnosing sensitive tissues or biology for protein identification.įace detection, which is an effortless task for humans, is complex to perform on machines. Interfacing these imaging techniques with our quantum pattern recognition processor provides input images that possess a better signal-to-noise ratio, lower exposures, and higher resolution, thus speeding up the machine learning process further. "interaction-free" imaging or "ghost" imaging. As an input to these pattern recognition algorithms, we consider experimental images obtained from quantum imaging techniques with correlated photons, e.g. The overall complexity of our pattern recognition algorithm is O(Nlog N) - $N$ is the image dimension. A novel quantum algorithm for finding dissimilarity in the faces based on the computation of trace and determinant of a matrix (image) is also proposed.
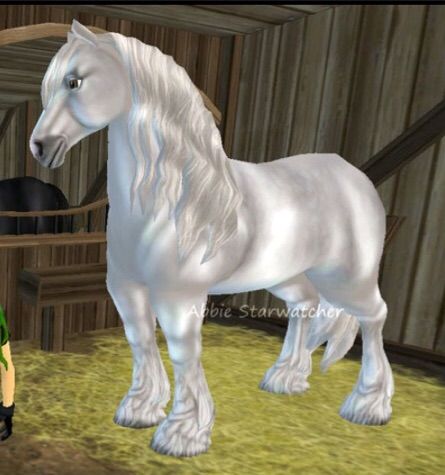
Here, we propose a quantum machine learning algorithm for pattern recognition based on quantum principal component analysis (QPCA), and quantum independent component analysis (QICA). Quantum algorithms have been shown to improve the efficiency and speed of many computational tasks, and as such, they could also potentially improve the complexity of the face recognition process. Pattern recognition with classical algorithms requires significant computational resources, especially when dealing with high-resolution images in an extensive database. Face recognition is one of the most ubiquitous examples of pattern recognition in machine learning, with numerous applications in security, access control, and law enforcement, among many others.
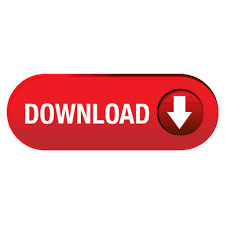